Evolution Strategies + Neural Networks = EVASION
Hallo!!
I found some weeks ago an article about Misfire Detection. It is from Ford Motor Company and can be read here: Aston Martin DB9 is High-Tech Under the Hood.
The Aston Martin DB09:
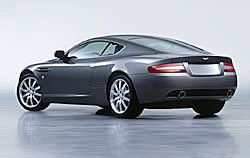
It is an interesting application of a Neural Network (NN) to help to solve a real world problem. The 2005 Aston Martin DB09 is the first Ford car with a Neural Network embedded to identify misfires. As some of you know, Neural Networks are tools that help to identify/detect patterns inside a set of data, these data can be random, noisy, etc, and some important patterns can occur so many times inside the data. But when a misfire occurs, also happens some disruptions of those patterns and the further pattern recognition can be difficult (or even incorrect), because "the misfires themselves may be isolated events, or they may form a pattern. That pattern is the signal we're looking for in all the noise and on a V-12, the frequency of firing events is so high that the legislative requirements for misfire detection could not be met with conventional computing resources. Neural networks offered us a whole new paradigm for computing and the potential for a misfire detection system that would be fully capable of meeting every detail of the regulations, something that the whole industry struggles with on any engine with eight or more cylinders", as explains Craig Stephens, manager of Research & Advanced Powertrain Controls at Ford Motor Co.
They could improve the Neural Network performance to identify patterns using Evolutionary Computation. An interesting method to to that is the EVASION Method. The EVASION Method uses an Evolution Strategy to optimize the Neural Network's structure (number of inputs, number of weights, number of neurons in the hidden layer, number of outputs, number of feedbacks and etc). "EVASION means EVAcuation out of the dimenSION. Valleys have to be formed at the edges of the optimization space (the zero weight axes), so that the gradient path is leading from the hyper-space to the adjacent hyper-subspace. Following the gradient-path evolution descends into the smallest possible subspace. Evolution-strategic learning will eliminate the superfluous weights.".
Below there is an Evolutionary Neural Network.
Generation 0 (Zero): At this generation the Neural Network is oversized and its performance is not so good.
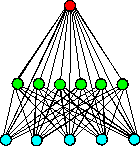
Generation 2000: This one is better than the above, but we can improve more its performance.

Final Compressed Neural Network: The best model found through the EVASION Method, its performance is much better than the other two above. I would be so much arrogant if I said that this final version is The Optimum structure for the Neural Network. Surely it is not, because that Neural Network could be very good to solve problems from a certain set, but it would be very bad to solve problems from other categories/types.
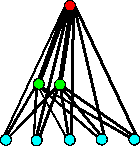
In the figures the thickness of the connections represents the weight strength of the Neural Network.
So, James Bond's Aston Martin would be much more intellingent and he could spend more time with girls and at ultra-exclusive parties. I am joking. :)
Just to remember the persons who visit my Blog: There are other methods and/or Evolutionary Algorithms (such as Genetic Algorithms, Evolutionary Programming and Genetic Programming) to evolve a Neural Network. Yes, Evolutionary Computation is much more than (only) Genetic Algorithm.
Até Mais!!
Marcelo
References:
http://www.bionik.tu-berlin.de/institut/xs2neuro
http://media.ford.com/newsroom/feature_display.cfm?release=18677
Ingo Rechenberg: Evolutionsstrategie '94. Stuttgart: Frommann-Holzboog 1994.
I found some weeks ago an article about Misfire Detection. It is from Ford Motor Company and can be read here: Aston Martin DB9 is High-Tech Under the Hood.
The Aston Martin DB09:
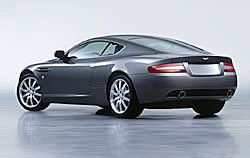
It is an interesting application of a Neural Network (NN) to help to solve a real world problem. The 2005 Aston Martin DB09 is the first Ford car with a Neural Network embedded to identify misfires. As some of you know, Neural Networks are tools that help to identify/detect patterns inside a set of data, these data can be random, noisy, etc, and some important patterns can occur so many times inside the data. But when a misfire occurs, also happens some disruptions of those patterns and the further pattern recognition can be difficult (or even incorrect), because "the misfires themselves may be isolated events, or they may form a pattern. That pattern is the signal we're looking for in all the noise and on a V-12, the frequency of firing events is so high that the legislative requirements for misfire detection could not be met with conventional computing resources. Neural networks offered us a whole new paradigm for computing and the potential for a misfire detection system that would be fully capable of meeting every detail of the regulations, something that the whole industry struggles with on any engine with eight or more cylinders", as explains Craig Stephens, manager of Research & Advanced Powertrain Controls at Ford Motor Co.
They could improve the Neural Network performance to identify patterns using Evolutionary Computation. An interesting method to to that is the EVASION Method. The EVASION Method uses an Evolution Strategy to optimize the Neural Network's structure (number of inputs, number of weights, number of neurons in the hidden layer, number of outputs, number of feedbacks and etc). "EVASION means EVAcuation out of the dimenSION. Valleys have to be formed at the edges of the optimization space (the zero weight axes), so that the gradient path is leading from the hyper-space to the adjacent hyper-subspace. Following the gradient-path evolution descends into the smallest possible subspace. Evolution-strategic learning will eliminate the superfluous weights.".
Below there is an Evolutionary Neural Network.
Generation 0 (Zero): At this generation the Neural Network is oversized and its performance is not so good.
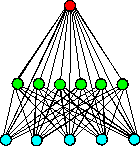
Generation 2000: This one is better than the above, but we can improve more its performance.

Final Compressed Neural Network: The best model found through the EVASION Method, its performance is much better than the other two above. I would be so much arrogant if I said that this final version is The Optimum structure for the Neural Network. Surely it is not, because that Neural Network could be very good to solve problems from a certain set, but it would be very bad to solve problems from other categories/types.
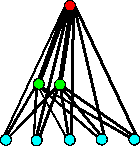
In the figures the thickness of the connections represents the weight strength of the Neural Network.
So, James Bond's Aston Martin would be much more intellingent and he could spend more time with girls and at ultra-exclusive parties. I am joking. :)
Just to remember the persons who visit my Blog: There are other methods and/or Evolutionary Algorithms (such as Genetic Algorithms, Evolutionary Programming and Genetic Programming) to evolve a Neural Network. Yes, Evolutionary Computation is much more than (only) Genetic Algorithm.
Até Mais!!
Marcelo
References:
http://www.bionik.tu-berlin.de/institut/xs2neuro
http://media.ford.com/newsroom/feature_display.cfm?release=18677
Ingo Rechenberg: Evolutionsstrategie '94. Stuttgart: Frommann-Holzboog 1994.